Joined up data in government: the future of data linking methods
Updated 16 July 2021
Foreword from the National Statistician, Professor Sir Ian Diamond
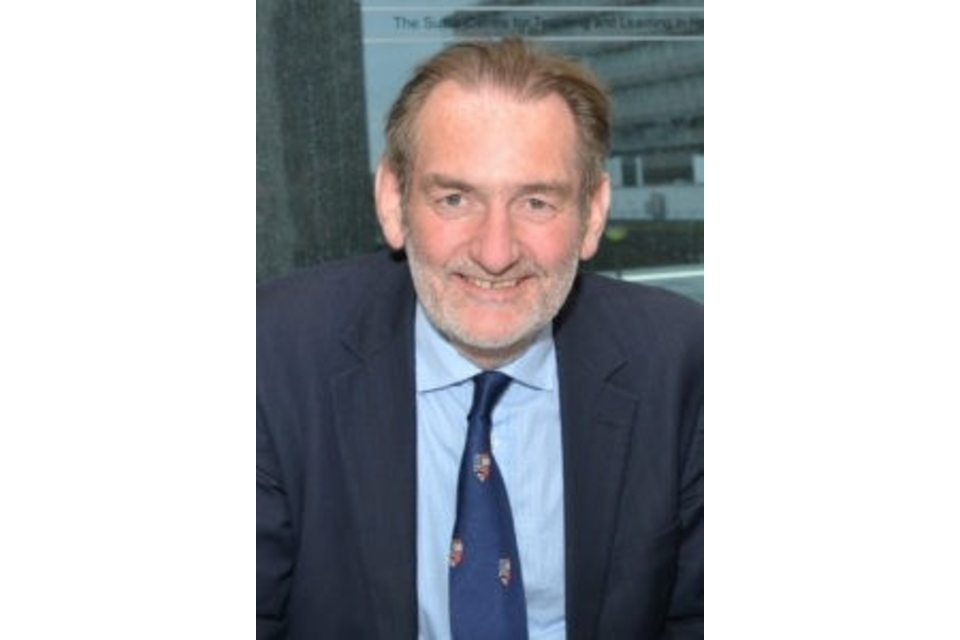
Professor Sir Ian Diamond
We find ourselves living in a society which is rich with data and the opportunities that comes with this. Yet, when disconnected, this data is limited in its usefulness. However, with the introduction of legislation such as the Digital Economy Act along with technical and methodological advances, we have ever increasing opportunities to link together this data and enrich the insights provided. Being able to link data will be vital for enhancing our understanding of society, driving policy change for greater public good and minimising respondent burden.
We must ensure that the data linkage methods we are using are in-keeping with best practice and evolving research trends across industries and identify skills or resource gaps preventing this. We also need to understand the potential of methods for linking anonymised data to understand the balance between maintaining data privacy and the utility of the data.
I welcome the findings from this review to improve work in data linkage across government. The recommendations made will give direction to important developments in building capability for all working on this essential component of government analysis.
Acknowledgements
We are grateful to have had the opportunity to work with leading experts from across government, academia, the third sector and internationally, thus ensuring the widest range of views on data linkage. This review faced adversity and was published during a particularly busy time for the Office for National Statistics (ONS). This wouldn’t have been possible without the hard work and support of the many people who contributed. In particular we would like to thank colleagues Rachel Shipsey, Josie Plachta and Shelley Gammon for providing their expertise and advice towards this review.
We dedicate this review to the memory of the late Harvey Goldstein, Professor at the University of Bristol and University College London (UCL), who sadly passed away on 9 April 2020. Harvey contributed significantly to this review with his novel scaling method for linkage. His wealth of experience in this field greatly helped inform the review’s recommendations to improve government linkage. We would also like to thank Katie Harron for her work to prepare Harvey’s article for publication, enabling us to share his work to help improve data linkage methods and application across government.
Brief summary
Why we did this review
Data and Analysis Method Reviews take topics of interest and innovation in data, review state-of-the-art methods and make recommendations for government work.
Data linkage provides insight, informs policy change and helps answer society’s most important questions through increasing the utility of data. It is integral to government operations, decision making and statistics. However, linkage presents challenges, as discussed in the Office for Statistics Regulation (OSR) 2018 report on joining up data, and more work needs to be done to realise its full benefits.
This review set out to engage with the data linkage community across government, academia, the third sector and internationally to understand challenges faced and identify state-of-the-art data linking methods to help realise those benefits.
What we found
The challenges we found include: how to assess the quality of linkage, how to link data effectively while maintaining privacy, and how to overcome the siloed nature of government linkage work.
We then commissioned a series of articles from recognised experts from academia, government and the third sector. These include state-of-the-art methods on:
- linking with anonymised data
- quality assessment of data linkage
- a framework for longitudinal linkage
- software for linkage
These articles have been peer reviewed by leading data linkage experts and published with this report.
Recommendations
The findings of this review led to a set of recommendations which focus on improving data linkage methods and capability across government. These recommendations will ensure there is strong investment in the field.
Developing cross-government data linking networks and increased collaboration with academia forms a large part of the recommendations. It is evident that across government departments there is considerable variation in data linkage skills and application, some being far more advanced than others. The review also identified a strong need for a more cohesive data linkage community, including better coordination of linkage projects, avoiding duplication of work, and sharing best practice for data linkage methods.
The review raised several new research areas where investigation into data linkage methods is needed to understand their potential. If successful, these new methods will improve data linkage, ensuring government keeps pace with new data sources and technology.
To ensure these recommendations are effective, they need support and adoption across all analytical professions and departments. An implementation plan for these recommendations will follow.
The recommendations of the review are to:
- build capability across government, including expanding the toolkit of data linkage courses, case studies and guidance
- improve collaboration across government, academia and internationally, including setting up a data linking network and organising linkage events
- conduct research on methods for Privacy-Preserving Record Linkage (PPRL), whilst carrying out linkage in-the-clear where possible to maintain quality
- work with networks to develop and maintain a quality culture when linking data, ensuring that quality metrics are produced and communicated to others
- conduct research on longitudinal linkage methods and quality metrics to understand how error progresses through the process and how to improve the linkage quality
- conduct research on the scaling method and compare with other linkage methods using large data sets
- conduct research on machine learning methods and their potential for government linkage, in terms of suitability and practicalities
- conduct research on scalable software solutions suitable for linking large data sets both within and across government departments, including Splink
- conduct research on graph databases for management of linked data sets
- explore options for producing test data for government and academia to test linkage methods. This includes new linkage algorithms and privacy-preserving techniques
Introduction
This cross-government review is part of a series, known as Data and Analysis Method Reviews. These cover topics of national importance and are sponsored by Professor Sir Ian Diamond, as head of the Analysis Function. These reviews are future facing, ensuring that methods used by the Analysis Function are keeping pace with changing theory, data sources and technologies.
Data linkage has never been more important for our society. Carrying out essential operations, making decisions that affect the country, and producing quality statistics to provide insights often rely on linked data. The methods used and quality of this linkage is crucial for ensuring the decisions made using this data are reliable and valid.
There is more opportunity than ever to link data together. The Digital Economy Act 2017 enables better sharing and use of data across organisational boundaries, at a time of dramatic increase in the range of sources and volume of data available. When disconnected, this data is limited in its usefulness. However, by linking data together we combine their resources and enrich the insights they can provide. Thus, enhancing our understanding of society, driving policy change for greater public good and minimising respondent burden.
There is significant data linkage work taking place across government to inform policy and provide greater insights while maximising the utility of collected data. A recent example of the importance of linking data was highlighted during the coronavirus (COVID-19) pandemic. Linking data from across government enabled rapid insights and ensured evidenced based decision making. For example, the lack of ethnicity information on death registrations was overcome by linking death registrations with the 2011 Census. This allowed for further research into the effects of the coronavirus (COVID-19) pandemic on different ethnic groups.
OSR’s 2018 review Joining up Data for Better Statistics provided a crucial insight into government data linkage work and future opportunities. Many positives were noted, but acknowledgement was given that greater work is needed to improve this field. Analysts across government should be encouraged to make data linkage a core part of their efforts to innovate and improve official statistics production.
Whilst there is a lot of data linkage taking place across government, this is often conducted in isolation with limited knowledge sharing. There needs to be a joined-up approach to ensure that data linkage is at the heart of improvements to official statistics. Furthermore, UK government linkage is falling behind other countries, especially those that have population registers and where ID numbers can be used for linkage. Therefore, time and investment are required for optimising and applying data linkage methods and ensuring that government has the skills required to link data optimally.
This review was conducted to identify state-of-the-art data linkage methods and to ensure a joined-up approach to improving data linkage occurs across government.
What is data linkage?
Data linkage (also known as matching, entity resolution or record linkage) is the process of joining data sets through deciding whether two records, in the same or different data sets, belong to the same entity (Harron et al., 2016). Records refer to entities such as events, people, addresses, households or businesses.
Examples of data linkage
Data linkage is an important process in analysis and is often conducted across government departments. For example, The Ministry of Justice (MoJ)/Department for Eduation (DfE) share provides data on childhood characteristics, educational outcomes and (re)-offending. This data share includes 20 DfE datasets, including data on academic achievement, pupil absence and pupil exclusions. It also includes 11 MoJ datasets, including data on offenders’ criminal histories, court appearances and time in prison. Each dataset has a unique ID variable that can be used to link across the datasets.
This linkage work has been undertaken for the purpose of increasing understanding of the links between childhood characteristics, education outcomes and (re)-offending. Sharing this data with accredited researchers will help develop the evidence base on understanding the relationships between educational and criminal justice outcomes and the drivers of offending. It will assist in identifying the population that requires support through early intervention and evaluating these projects to understand whether they are effective.
Data linkage’s utility also extends outside of government, for example playing an important role in business analysis. This includes combining data sources on customers, employees, financials and operations to uncover relationships among important variables. These relationships can reveal insights into customer feedback, operational and employee metrics and more.
Data linkage challenges
Data linkage is a complex field that can involve lots of different parties and intricate methodologies. From our engagement, we identified important challenges in government linkage methods and application to address:
- There are different software, skill levels and resources across departments, meaning varied linkage capability in government.
- Siloed working between departments can make it difficult to share research and good practice across government.
- There is a lack of commonly used open source software tools for linkage.
- It is difficult to understand the effects of data anonymisation on linkage quality.
- Departments show varied understanding of how to measure, interpret and communicate linkage quality.
- Changes in data records over time make longitudinal linkage (linking data across time) challenging.
What is included in this review?
This review contains a series of contributed articles, on state-of-the-art data linkage methods and applications from recognised experts. These state-of-the-art methods have been chosen due to their potential applicability and as a springboard to improving data linkage across government. The contributing articles have been peer reviewed by leading data linkage experts to validate the methods and to evaluate their suitably for application across government.
After conversing with experts and engaging with government on important linkage areas, the following contributed article topics were agreed:
- methods for linkage, including linking with anonymised data
- quality assessment of linkage
- a framework for designing longitudinal linkage
- software for linkage
This review contains a set of recommendations concentrated on improving data linkage methods and capability across government. These recommendations will ensure that there is strong investment in the field. They have been developed from the contributed articles, the data linking challenges currently experienced across government and engagement with experts.
While this review mentions challenges in accessing data and data sharing, this is not in the scope of the methods review.
Emerging themes and recommendations
The main findings from the contributed articles and stakeholder engagement during this review have been grouped into themes, along with recommendations for government linkage work. These recommendations will form part of a cross-government data linkage programme to improve linkage work over the next few years. An implementation plan, for the recommendations, will be published later in 2020.
1. The data linkage community need to work together to improve methods, their application and skills
Government carries out a wide range of data linkage work to meet many different needs. Increased collaboration between departments and professions, as well as academia, the third sector and international bodies, will be essential for maximising the quality of government linkage and sharing good practice, as well as building capability for all involved in linkage work.
It is evident that across government departments there is variation in data linkage skills and application, some being far more mature than others. We need to work together to improve data linkage, avoid working in silos and ensure no department is left behind.
We discuss some crucial areas for improving collaboration and capability.
Data linking community
Successful data linkage inevitably requires departments to work together. Following the data linking symposium held in October 2019 and our stakeholder engagement, it is clear that government want to do more to work together and understand the data linkage work happening across departments. Through these discussions and suggestions from the contributed articles, we identified areas that may benefit from a more collaborative community.
Having a network of data linkage representatives across government would further sharing of cross-government projects, to link up with relevant areas and avoid duplication of work. A network would also allow departments to share good practice more effectively, for example case studies, to help other departments build capability. We plan to model this network on the quality champions network, which has proven successful in building capability and sharing knowledge. We also plan to engage with senior leaders across departments to gain their buy-in and ensure resource can be devoted to improving data linkage across government.
We are also seeing increased data linkage engagement in the online community on platforms such as Slack. This would also be an ideal forum to organise and promote events, such as online webinars showcasing linkage work and face to face events. For example, the Best Practice and Impact division (BPI) at ONS previously ran a sharing webinar on data linkage where presenters shared successful linkage projects, showcasing good practice to the linkage community.
Engagement outside of UK government is also beneficial for linkage work. Projects, such as this review, have benefitted hugely from academic and international collaboration. Academics are fundamental to the development of new methods for linkage and working with them to test methods on government data will be an important step for operationalising these methods for government use. We will encourage increased collaboration and engagement with external leading experts.
Skills
There are several ways we can work to build linkage capability across government. Increasing the availability of different levels of training will help ensure colleagues are equipped with skills to perform linkage.
Discussions at the 2019 data linking symposium showed people wanted more guidance on data linkage. This included guidance on linkage quality and how to transition to more sophisticated data linkage methods. Some departments have written their own tailored data linkage guidance, and this would benefit from a joined-up approach. This could include published case studies and guidance.
As well as analytical professions, we will also increase awareness of usage and application of linkage methods across other professions such as policy.
Recommendations
- Build capability across government, including expanding the toolkit of data linkage courses, case studies and guidance.
- Improve collaboration across government, academia, the third sector and internationally, including setting up a data linking network and organising linkage events.
2. There is a trade-off between linkage quality and maintaining privacy
The trade-off
Methods for data linkage are optimised for in-the-clear (readable, unencrypted) data. Shipsey and Plachta make the case for using in-the-clear data for linkage wherever possible; linking data sets with visible Personally Identifiable Information (PII) allows for better quality assurance of methods and clerical review to resolve matches not made by the linkage algorithm, both of which improve overall linkage quality. Schnell also discusses the advantages of in-the-clear linkage, including being able to clerically review non-matches, quicker process time, and the ability to making changes to procedures as more data becomes available. Linking data in-the-clear also requires far less resources than linking anonymised data.
To protect privacy of entities in linkage, data can be hashed using mathematical algorithms that turn data into fixed-length strings of digits and characters for enhanced security. Whilst this preserves the privacy of data records, when data is securely hashed there is no longer any relationship between similar values (for example, Jon and Jonathan), meaning these similarities cannot be used when linking records. Additionally, clerical review cannot be done to check the quality of the linkage.
This trade-off between maintaining privacy of entities and linkage quality is a challenge faced by government departments. It will be important in the future to coordinate with projects aiming to make improvements in this area.
Emerging methods in Privacy-Preserving Record Linkage
Schnell describes leading methods of PPRL and the advantages and disadvantages of these methods. He also discusses the need to develop agreement on what scenarios privacy-preserving methods are trying to prevent.
Shipsey and Plachta discuss the challenges of PPRL further and outline a new method, called Derive and Conquer, for linking hashed data. The next step with this work is to quality assure the method with samples of in-the-clear data to understand the quality of the linkage compared with in-the-clear matching. They recommend that wherever possible, data acquisition teams should negotiate to receive data in-the-clear. However, when this is not possible, departments should devote resource to supporting the process-heavy running of hashed data linkage algorithms. Also, samples of in-the-clear data must be made available to check the quality of the linkage.
Recommendation
- Conduct research on methods for PPRL, whilst carrying out linkage in-the-clear where possible to maintain quality.
3. Understanding the quality of linkage is essential
Understanding linkage quality is important for appropriate use of linked data. Linkage error can have many adverse consequences, including:
- missing or incorrect data for analysis
- mistaken inclusion or exclusion of records from a data set
- records that are split to look like multiple records
- merging of multiple records to appear as one entity
Linkage error can affect analysis and interpretation of data, leading to incorrect conclusions and misuse of data.
Some level of linkage error is unavoidable, particularly when working with data sets of variable data quality. However, understanding this error allows users to account for this in their analysis or operational processes.
In terms of measuring linkage quality, it is about much more than the match rate (the proportion of records that have been linked). Whilst there is some good practice with reporting linkage quality in government, there is work to be done to ensure linkage error reporting is integrated into processes and there is clear communication about linkage quality to users.
Doidge, Harron and Christen discuss the different quality metrics available, including what level of information is needed for the different approaches, as well how to communicate this to users (more information is given in table 1). They also highlight that whilst there are privacy advantages to the separation often found between data linkers (those responsible for linking the data) and data analysts (those who use the data after linkage is complete), there are things we can do to bridge this gap and get the most out of linked data. The authors make a series of recommendations for data linkers and data analysts to improve the quality of linkage work.
Identifier needed? | Technique | Required inputs | Potential outputs |
---|---|---|---|
Yes | Training data (gold standard) | Identifiers | Rates of missed links and false links and/or distribution of error rates |
Yes | Clerical review | Identifiers +/- supplementary matching data | Human estimation of match status leading to estimated rates of false links and distribution of false links |
Yes | Negative controls | A set of records not expected to link that can be submitted to the linkage procedure | Rates of false links |
No | Unlikely or implausible links | Links excluded by data linkers during quality assurance; and/or records with multiple candidate links even when only one is possible; and/or payload data | Distribution of false links, rate of false links* |
No | Analysis of matching variable quality | Record-level or aggregate indicators of matching variable quality | Identification of unlinkable records Distribution of missed links and/or likely missed links |
No | Comparison of linked vs unlinked records | Unlinked records, or aggregate characteristics of unlinked records, when all records in one or both files are expected to have matches | Distribution of missed links Rate of missed links when expected match rate = 100%, given rate of false links can also be estimated |
No | Positive controls | Unlinked records for a subset of records expected to have matches | Distribution of missed links Rate of missed links, when rate of false links can be estimated |
No | Comparison of linked data to external reference statistics | Statistics derived from another representative data set for observable characteristics of the linked data | Rate of missed links* Rate of false links* Distribution of missed links* Distribution of false links* |
Table 1: Techniques for linkage quality assessment recommended by Doidge et al., separated by those that require access to unique identifiers and those that don’t, which are recommended for data linkers to report on to data users.
*The term ‘rate’ is used very loosely in this table to refer to any measurement of errors, and some outputs depend on the context.
There also isn’t a one size fits all approach to linkage; it is important to tailor linkage work so the outputs fit user requirements. Users will have different requirements for quality depending on the data need. For example, if linking is used to identify and inform people who have been exposed to something dangerous, it is more important to identify every possible match. This may come at the cost of having some false matches. However, in other circumstances accuracy of links may be the priority, for instance if linkage is being used to discuss a sensitive health issue. Doidge et al. discuss this in more detail.
Blackwell and Rogers discuss how to gather user requirements for linkage. This is crucial as data quality requirements can differ for operational purposes and statistical purposes. Data linkers should understand the quality requirements of data users prior to linkage and build this into the linkage work. Users should also engage with data linkers to understand their approach and discuss expectations of linkage quality and outputs.
Recommendation
- Work with networks to develop and maintain a quality culture when linking data, ensuring that quality metrics are produced and communicated to others.
4. Design is important when linking longitudinal data
Longitudinal data linkage opens up opportunities for maximising the utility of data over time. For instance, it can be used to build an understanding of population changes over time by linking different censuses. This reduces costs associated with additional data collection methods (such as surveys) and reducing the burden on respondents to provide data that is already available elsewhere.
However, there are a lot of potential sources of error during the data journey that need to be understood to ensure longitudinal linkage is fit for purpose. Blackwell and Rogers discuss considerations to make when linking longitudinal data to meet user needs, in the context of using longitudinal linkage to estimate international migration. It is important to understand the design of administrative data and the error at each stage of the process to build this into the linkage design. They have designed a longitudinal linkage error framework for use when linking for statistical purposes. They recommend it is used to identify error sources in the data journey to build into statistical design and develop quality indicators for reporting on the statistical properties of the linked data set.
Recommendation
- Conduct research on longitudinal linkage methods and quality metrics to understand how error progresses through the process and how to improve the linkage quality.
5. Further research is needed to develop and operationalise methods
This review has raised several areas where more research into linkage methods is needed to understand their utility. These methods have tested well on small-scale data sets. However, further research is needed to assess whether they are applicable to large-scale government data.
Linkage methods
Goldstein’s work discusses a novel linkage method that may aid the ease of linkage across government. This method adopts a scaling algorithm based on correspondence analysis, and potentially offers an alternative to traditional probabilistic methods that is more computationally efficient and intuitive for users. To date this method has only been tested on small data sets; access to real data for testing is fundamental for government to improve understanding and consider implementation of this method.
Doidge et al. outline the potential of machine learning methods for improving discrimination between records and applications of this, for instance clustering groups of records (such as members of the same household). This is an area that has not been explored fully using large-scale data sets and needs further research to understand how this can apply to government data.
Software
Different software is used across government to link data. This can cause difficulties when coordinating linkage, both across departments and different systems within departments. Additionally, most open source software is not suitable for linking millions of records - a requirement for many government linkage projects. Linacre describes Splink, the Ministry of Justice’s in-house open source software solution for linkage. This is an application of the expectation-maximisation algorithm to the Fellegi-Sunter linkage model, run on Apache Spark. They discuss the improvements Splink offers over other packages. The package has tested well on data sets containing 15 million records. Such software needs further testing to find solutions suitable for large-scale government linkage.
Test data
A lot of the research described in this review needs test data (data that represents the population of interest for testing the effectiveness of processes or computer programs). These include:
- Goldstein’s scaling algorithm (this requires access to real data for testing)
- the machine learning methods described in Doidge et al. (their success depends on the quality of the data, the amount of computing resource and expertise available)
- test data for the academic community to develop and test PPRL approaches using data that can simulate real life data problems (discussed in Schnell)
- testing linkage algorithm quality when applied to hashed data (discussed in Shipsey and Plachta)
Government need to look at how we can move forward with this research and best employ test data.
Data management
Graph databases have recently shown promise as a method for storing and processing data in linkage projects. This allows data linkers to store relationships between records in the database, maintaining knowledge of their potential links. This knowledge can inform subsequent linkage when more data is added or changed. Another benefit is this could increase linkage quality without requiring expensive clerical review. Graph databases are a new approach for linkage projects and further research is needed to understand its robustness and utility in government.
Recommendations
- Conduct research on the scaling method and compare with other linkage methods using large data sets.
- Conduct research on machine learning methods and their potential for government linkage, in terms of suitability and practicalities.
- Conduct research on scalable software solutions suitable for linking large data sets both within and across government departments, including Splink.
- Conduct research on graph databases for management of linked data sets.
- Explore options for producing test data for government and academia to test linkage methods. This includes new linkage algorithms and privacy-preserving techniques.
Implementation of recommendations
To implement the recommendations in this review, the Government Data Quality Hub, within ONS will:
- build new data linking networks and work with existing networks
- work with already established projects across the analysis function to action the recommendations from this review
- contact experts in government to scope additional required work and create an implementation plan for the recommendations
The Government Data Quality Hub will publish an implementation plan and report every six months on the progress of these recommendations. These will be published on the gov.uk analysis function website.
Contributed articles
Quality assessment in data linkage
About the authors
Dr James Doidge, Intensive Care National Audit & Research Centre
James has been living and breathing data linkage for the last 9 years. This was first as a researcher establishing population-level studies of linked administrative data in Australia and the UK, then as a data linker at Public Health England and the Intensive Care National Audit and Research Centre (ICNARC), and through advisory roles with ONS and NHS Digital. James is a methodologist with experience in a wide range of research fields, including medicine and healthcare, nutrition, child protection and education. His current role focuses on the design of efficient clinical trials and observational studies that maximise the use of routinely collected health data.
Dr Katie Harron, University College London
Katie is an Associate Professor at the UCL Great Ormond Street Institute of Child Health. She completed her PhD on statistical methods for data linkage at UCL in 2014 and was subsequently awarded a Wellcome Trust fellowship. Katie’s research uses statistical methods to exploit the rich data that are collected about populations as we interact with health, social care, and educational services throughout our lives. She is particularly interested in the complexities of linking large data sets, evaluation of linkage quality, and the use of electronic healthcare data to support clinical trials. She is passionate about increasing the public understanding of using administrative data for research.
Prof Peter Christen, Australia National University
Peter is a Professor at the Research School of Computer Science at Australia National University (ANU). His research interests are in data mining and record linkage, with a focus on machine learning and privacy-preserving techniques for record linkage. He has published over 150 articles in these areas, including in 2012 the book “Data Matching”. He is a co-author of the forthcoming book “Linking Sensitive Data” (Springer, 2020). Peter is the principle developer of the Freely Extensible Biomedical Record Linkage (FEBRL) open source data cleaning, deduplication and record linkage system. He has served on the program committees of various data mining conferences and workshops, has been on the organisation committee for the Australasian Data Mining conferences since 2006, and co-organised the workshops on Data Integration and Applications since 2014. He has also served as reviewer for a variety of top-tier international journals, and as assessor for the Australian, UK, and Canadian Research Councils. He is also involved in the Economic and Social Research Council (ESRC)-funded Digitising Scotland project, which aims to construct linked genealogy of Scottish historical records.
Longitudinal linkage of administrative data; design principles and the total error framework
About the authors
Dr Louisa Blackwell, Office for National Statistics
Louisa is a Principal Statistical Methodologist in ONS Methodology, leading on demographic methods. Her PhD in Social Statistics (City University) was on occupational segregation and part-time work and involved modelling linked administrative data from the ONS longitudinal Study. Her career has spanned academic and government research. She was Principal Researcher on the ONS Longitudinal Study. For the 2011 Census she was Data Quality Manager and led on Census/ Administrative Data Matching. Currently she is leading the development of measures of statistical uncertainty for ONS population estimates in collaboration with the University of Southampton, developing a new admin-based cohort study jointly with the Home Office and she is a member of the United Nations Economic Commission for Europe (UNECE) Task Force on the Use of Longitudinal Data for Measuring International Migration.
Nicola Rogers, Office for National Statistics
Nicola is a Principal Statistical Methodologist in ONS Methodology, leading on demographic methods. Her MSc in Official Statistics (University of Southampton) dissertation was on ‘The Effects of individuals and place as predictors of list inflation in the NHS Patient Register’ and involved multi-level modelling of linked Census/ administrative data. Her career includes social research in government, academia and local authorities. She has expertise in Small Area Estimation, the ONS Longitudinal Study, Administrative Data Matching for the 2011 Census and the Improving Migration Statistics Programme. Currently she is leading ONS secondments to the Home Office to advance the statistical use of Exit Checks data and developing new methods for measuring international migration, together with associated measures of statistical uncertainty. She has also chaired work sessions of the UNECE Task Force on the Use of Longitudinal Data for Measuring International Migration.
Privacy-Preserving Record Linkage in the context of a National Statistics Institute
About the author
Prof Rainer Schnell, University of Duisburg-Essen
Rainer holds the chair for Research Methodology in Social Sciences at the University of Duisburg-Essen, Germany. From 2015 to 2017 he was the Director of the Centre for Comparative Surveys at City, University London. He was the founding editor (2006 to 2013) of “Survey Research Methods”, the methodology journal of the European Survey Research Association. Rainer founded the Centre of Quantitative Methods at the University of Constance and the German Record Linkage Centre. His research focuses on non-sampling errors, applied sampling, census operations and PPRL.
Linking with anonymised data – how not to make a hash of it
About the authors
Dr Rachel Shipsey, Office for National Statistics
Rachel works as an operational researcher in data linkage methodology at the Office for National Statistics. She is currently project lead for 2021 Census linkage and linking with encrypted data projects. Her background is in algorithmic number theory with applications in cryptography and she has previously worked as an academic researcher and in education. Current work includes the development of optimised deterministic and probabilistic matching algorithms, machine learning in data linkage, algorithms that enable efficient calculation and parallelisation of processes, understanding the limitations of linking with encrypted data and explainable Artificial Intelligence.
Josie Plachta, Office for National Statistics
Josie Plachta works as a methodologist with the UK Office for National Statistics, specialising in data linkage. She is currently developing the derive and conquer hashed matching algorithm, as well as researching census linkage using deterministic and probabilistic methods, and current NHS linkage projects. Her background is in evolutionary biology and zoology, and has previously worked in the NHS, specialising in Addiction Recovery Clinic data.
Efficient procedures for linking data sets for the purpose of fitting statistical models
About the author
Prof Harvey Goldstein, University of Bristol/University College London
Harvey Goldstein was an esteemed professor at the University of Bristol and UCL. A statistician and social scientist, he had a long, varied career and was highly influential in the statistics community. He is particularly well known for his work on multilevel modelling, and he founded the Centre for Multilevel Modelling (CMM) that now sits within the University of Bristol. He advised government departments throughout his career and contributed his expertise to government projects such as this review.
Information for this biography was taken from an obituary written by his colleagues at the University of Bristol, which gives more details on his life’s work.
Splink: MoJ’s open source library for probabilistic record linkage at scale
About the author
Robin Linacre, Ministry of Justice
Robin is a data scientist leading work on data linking methodology at the MoJ. He has a background in econometrics but more recently has worked on a variety of open source analytical packages and infrastructure. In his previous role, he worked on the MoJ’s new analytical platform, designing the data engineering infrastructure to enable analysts to rapidly perform analysis on big data sets.
Contact and resources
For any queries or to find out more about this review please contact [email protected].
For explanations of specialist terms, refer to the accompanying glossary. Also, for more resources on data linkage, please see the related guidance page on the Government Statistical Service website.